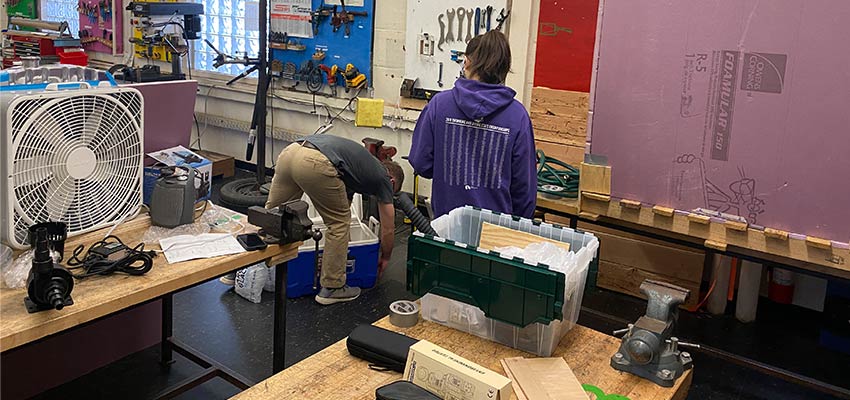
Increasing the effectiveness of GeoAirCon’s geothermal cooling system in Pakistan through physical experimentation, machine learning modeling, and heat transfer simulations to identify key parameters such as water and air flow rates, with the goal of informing GeoAirCon's system design to better serve over 1 billion people lacking affordable cooling solutions.
MIT D-Lab class
Community partners
- GeoAirCon
- Muhammad Hassamuddin, CEO
Country
Pakistan
Student team
- Matt Hoel- SM Mechanical Engineering & MBA, 2026
- Ya Qin- Harvard Design Studies (Ecologies), 2025
- Kathy Zhao- Mechanical Engineering, 2026
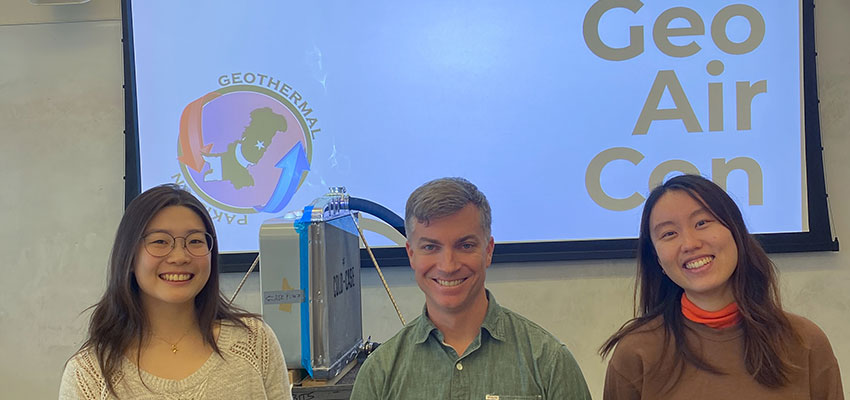
Cultural and/or market context
Across India and Pakistan, climate change is driving extreme heat waves with temperatures often exceeding 45 degrees Celsius. While demand for air conditioning is projected to triple by 2050, over 80% of households in these regions—representing more than one billion people—cannot afford it due to high upfront and operating costs. This lack of access is contributing to rising heat-related illnesses and fatalities, resulting in 568 deaths recorded in Pakistan over just six days in June 2024. As extreme conditions worsen, the need for affordable, sustainable cooling solutions is becoming ever more critical.
Problem framing
In response to this growing challenge, GeoAirCon has developed an affordable climate control system using geothermal cooling. However, they are sometimes unable to reach a desired indoor temperature range of 22 ± 4°C in extreme temperatures. Our team used physical experimentation and heat transfer simulations to vary system parameters in order to understand how to reach this cooling target.
Competitive analysis
Compared to AC, GeoAirCon’s solution requires less electricity to run, resulting in lower operation costs and thus greater affordability. Compared to a fan, which is currently the most common alternative, GeoAirCon’s solution is more effective and actually lowers the temperature of indoor spaces, rather than relying on evaporative cooling.
Solution including technical details
Our team utilized physical experimentation, a machine learning model, and heat transfer simulations to vary system parameters and understand how to reach the cooling target. Through experimentation, it was determined that pump flow rate had the greatest impact on stabilized temperature, while fan speed had the most significant influence on the initial cooling rate. The machine learning model provided a tool for GeoAirCon to determine ideal starting conditions for further testing on their deployed systems with changing inputs. Simulations further supported that achieving the target temperature range is possible with the selected parameters, confirming the conclusions drawn from physical tests.
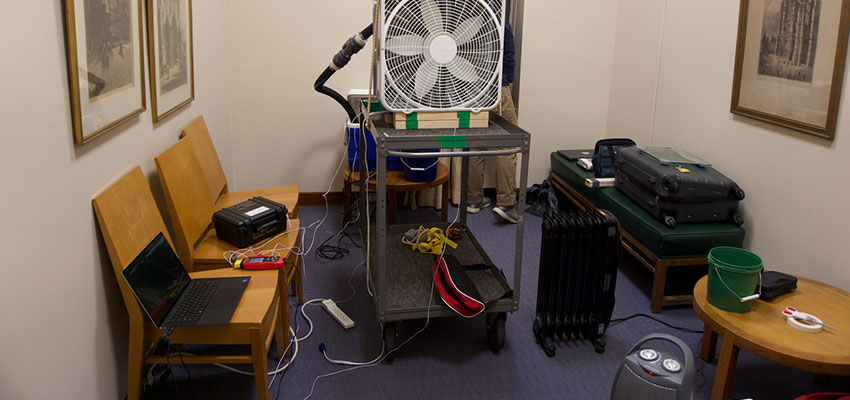
Hand-off to partner, next steps
We recommend testing radiators with varying geometries, fans, and configurations, using the data to refine predictive models like random forests. GeoAirCon should validate results by starting with low water and medium air flow rates, incrementally adjusting settings. Additionally, explore solutions like variable fan shrouds, reflective coatings, or preheating/cooling inlet water.
Contact
Macauley Kenney, D-Lab: Design for Scale Instructor
Mathieu Aguesse, D-Lab: Design for Scale Instructor